Advances in deep learning technology have generated today’s hype surrounding artificial intelligence. Applications such as ChatGPT and Lensa AI have captured the imagination of users worldwide. The tools are fascinating because they can create text, art and more, blurring the lines between machine and human capabilities.
Deep Learning: How It Works, How It’s Used and How It Can Create Risks
Deep Learning: How It Works, How It’s Used and How It Can Create Risks
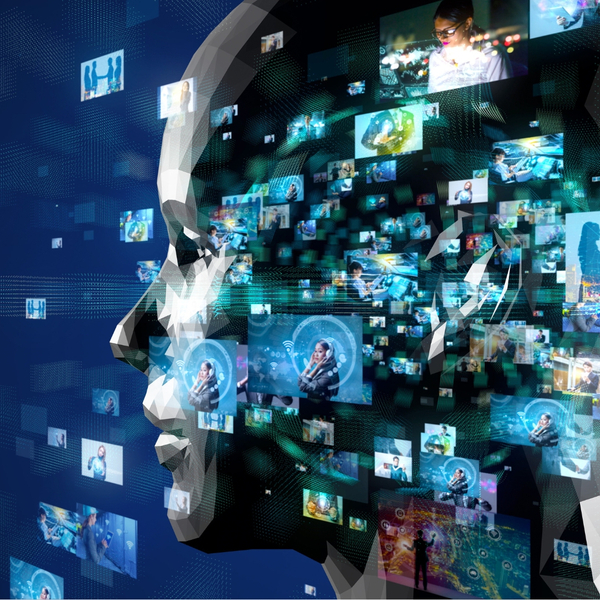
That’s what deep learning is all about. The neural networks at the heart of most deep learning algorithms simulate the activity of neurons in the human brain. The brain is a complex organ that, among other things, receives and interprets information from the senses. Neurons are the brain’s primary functional cells, creating thoughts, memories, movements, feelings and sensations as they pass information to each other.
Neural networks mimic this activity by processing data in layers. The input layer includes nodes that receive raw data, process it and send it to nodes in the next layer. This procedure is repeated until the data reaches the output layer, which produces the result. Traditional neural networks have four or five layers, but deep learning networks can have up to 150.
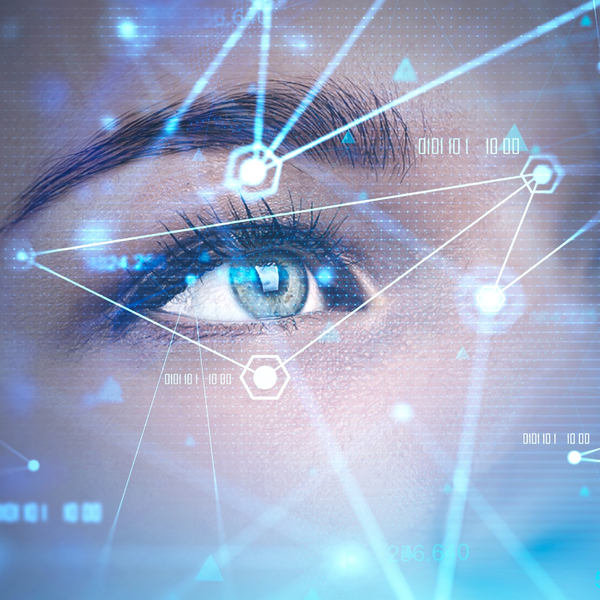
How Deep Learning Works
The deep learning concept dates to the 1980s, but the technology only became practical in recent years. Deep learning requires substantial compute power, which traditionally meant a supercomputer in a government agency, major university or research lab. Today, graphics processing units (GPUs) and other specialized chips provide the processing speed needed to train neural networks. Increasingly, this compute capacity is available in cloud-based platforms, along with other tools needed to design and train deep learning models.
Deep learning also needs a large amount of data for training. For example, the Stable Diffusion neural network that powers Lensa AI was trained using the Large-Scale Artificial Intelligence Open Network (LAION). LAION has a database of 5.85 billion multilingual image and text pairs. This enables Lensa AI to generate social media avatars in various art styles based on the user’s photo and textual inputs.
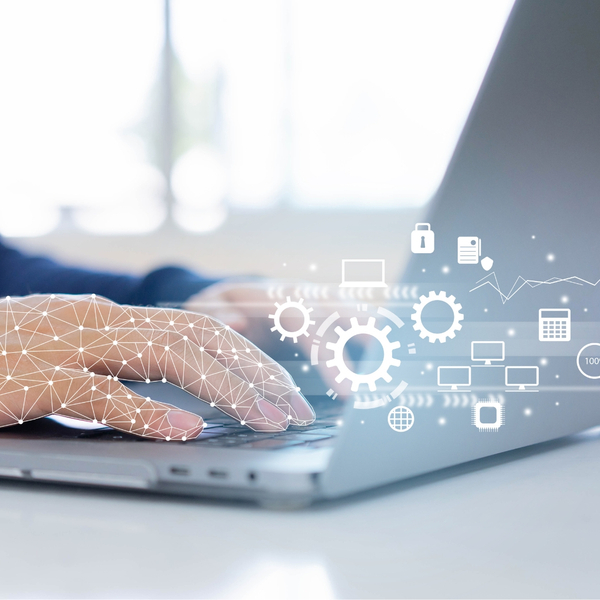
Deep Learning Use Cases
The larger the dataset, the better the deep learning model performs, and that’s a key distinction between deep learning and machine learning. “Shallow” machine learning models will ultimately reach a plateau, beyond which they do not improve with more training data. Deep learning models also learn to identify relevant characteristics in data automatically, while machine learning models are trained with data that has been tagged.
These characteristics make deep learning the best choice for use cases that require complex analysis. A primary application is in cybersecurity. Unlike traditional rules-based systems, deep learning tools can detect new types of security threats, analyze device and user behaviors, and even predict the potential for an attack.
Three industry-specific examples of deep learning applications include:
Fraud detection. Deep learning models can help financial services firms reduce risk and enhance customer trust by identifying fraudulent transactions.
Medical research. By analyzing medical images and other data, deep learning models can detect cancer in its early stages and aid in developing new treatment options.
Defense. Deep learning systems can help improve the safety of troop deployments by identifying objects in satellite imagery.
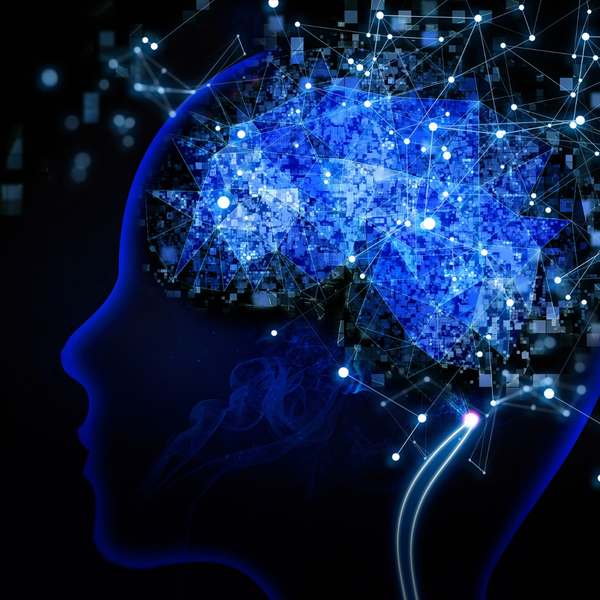
Deep Learning Risks
Deep learning applications are opening up new possibilities in business intelligence, but it’s not without risks. AI “hallucinations” are a very real problem. Hallucinations occur when the AI tool generates false information, contradicts itself or provides irrelevant output. They are typically the product of poor-quality training data. Bias in the deep learning model or training methods can cause hallucinations as well.
Hallucinations can also be the result of data poisoning attacks. Cybercriminals purposely inject bad information into the training data such that the model delivers inaccurate results.
To reduce these risks, organizations should not blindly follow the output of AI-enabled tools. While there’s a tendency to anthropomorphize AI, it’s important to remember that the technology does not know whether its output is correct. The basic accounting policy of “trust but verify” holds — ensure that the AI tool is using high-quality data that hasn’t been manipulated.
DeSeMa understands deep learning development and its practical applications. Let us help you take advantage of this technology while minimizing the potential risks.