The natural language processing market is booming. Researchers with Fortune Business Insights expect the NLP market to exceed $112 billion by 2030, a compound annual growth rate of 24.6 percent. Organizations are adopting NLP tools to increase the efficiency of business processes and capture more insight from large volumes of unstructured data.
Natural Language Processing Enables Efficiency and Better Decision-Making
Natural Language Processing Enables Efficiency and Better Decision-Making
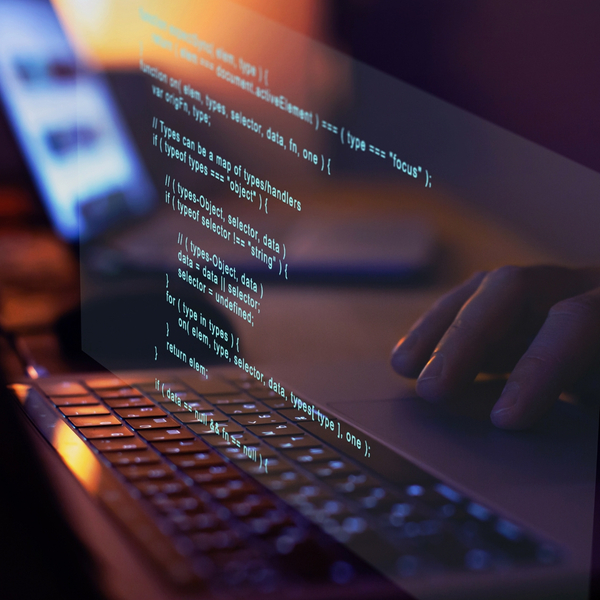
NLP is a type of artificial intelligence that gives computers the ability to understand spoken and textual communications. Some of the best-known NLP applications include virtual assistants, speech-to-text translators and similar tools that consumers encounter in their daily lives.
Microsoft Copilot is a notable example. This tool’s Business Chat feature can extract and summarize information relevant to the user’s natural language prompt from all the files in an organization’s Microsoft ecosystem. Other NLP tools are used to detect spam and malicious emails, and analyze intent, sentiment and urgency in customer communications.
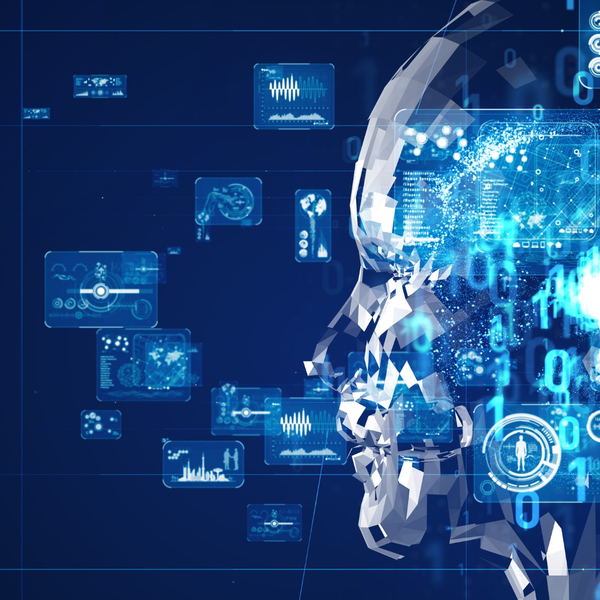
How Natural Language Processing Works
NLP uses deep learning, machine learning and statistical models, coupled with computational linguistics, to understand human language. This is no easy feat. NLP tools must learn not only grammar rules but exceptions and variations. Idioms, metaphors, sarcasm and many other irregularities add to the complexity. To be useful, the applications must be able to process this all accurately in real time.
Speech recognition adds another hurdle. The application must understand different accents and manners of speaking, even if the person talks quickly and slurs words together. It must then identify the part of speech and meaning of each word, recognize names, and more. It may also analyze the speaker’s sentiment to identify emotions, attitudes and other subjective qualities.
An NLP application’s capabilities depend on the type of algorithm used and how the system is trained. The simplest NLP applications are rules-based — they use systems of linguistic rules to understand text. For example, basic customer service chatbots that use decision trees or keywords have limited capabilities. Machine learning-based applications can answer open-ended questions and learn over time to improve their responses.
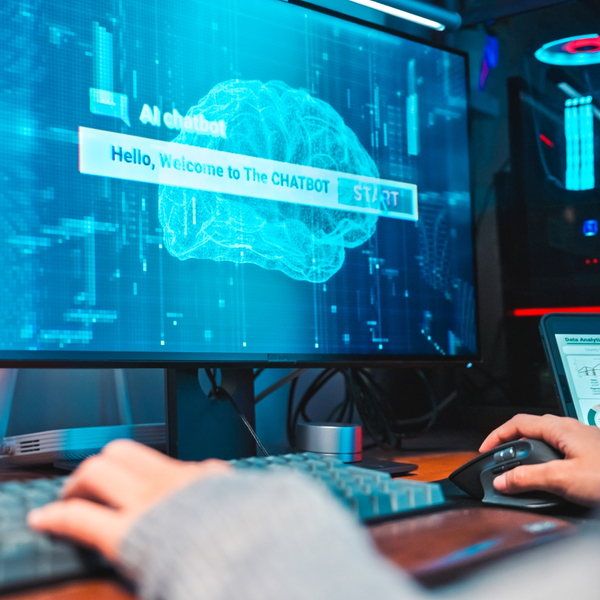
Natural Language Processing Examples
NLP applications trained using large datasets are the most advanced. Microsoft Copilot is based on GPT-4, OpenAI’s multimodal large language model, which was trained using 45GB of data. GPT-4 is even better than its predecessor at predicting what word should come next in a sentence and producing coherent-sounding output. GPT-4 scored in the 93rd percentile of the SAT and the 90th percentile of the Bar Exam, illustrating its ability to formulate correct-sounding answers. GPT-4 can also accept visual prompts, solve math problems and tell stories.
Advanced NLP tools are seeing widespread adoption, particularly in industry sectors such as healthcare and financial services. For example, much of the patient data entered into electronic health records is unstructured, such as physician’s notes, radiology reports and treatment plans. Much of this data goes unused because it’s difficult for humans to analyze. NLP applications can summarize this data to help clinicians make more effective diagnoses.
In financial services, NLP tools can analyze a company’s public filings and market history to offer insights into its financial performance. Sentiment analysis can assess the subjective qualities of earnings calls, financial news and social media posts.
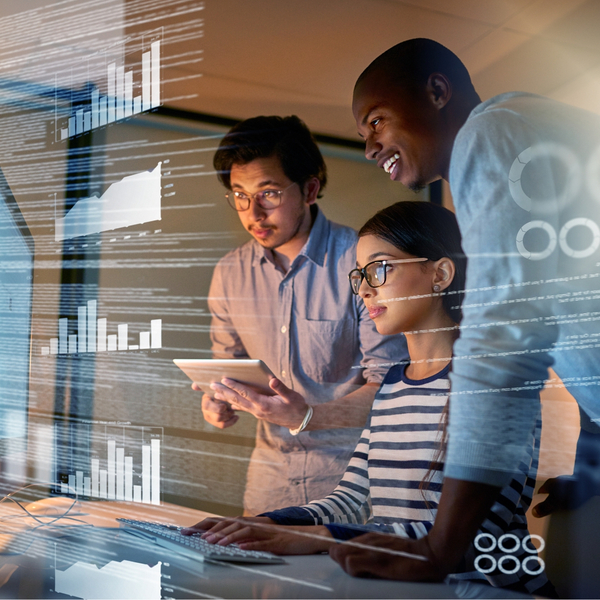
Preventing the Exposure of Sensitive Information
Organizations that implement NLP applications should take steps to protect sensitive information. This involves classifying data and implementing security controls that prevent the NLP tool from exposing data to individuals who shouldn’t access it.
Let’s say someone asks your chatbot, “Does XYZ Company use your systems?” The chatbot should be able to determine whether your organization has a marketing agreement with XYZ. If so, the chatbot could respond, “Yes, XYZ is a client and here’s a press release that describes what we do for them.” If not, the chatbot could say, “We don’t currently have a marketing agreement with XYZ.”
If you’re looking to implement NLP, DeSeMa can work with you to identify and classify sensitive information and what user roles are authorized to access it. Let us help you tap the power of NLP without creating security, privacy and regulatory compliance risks.